Artificial? Yes. Intelligent? No.
- lmohnani3479
- Oct 20, 2024
- 4 min read
Updated: Dec 22, 2024
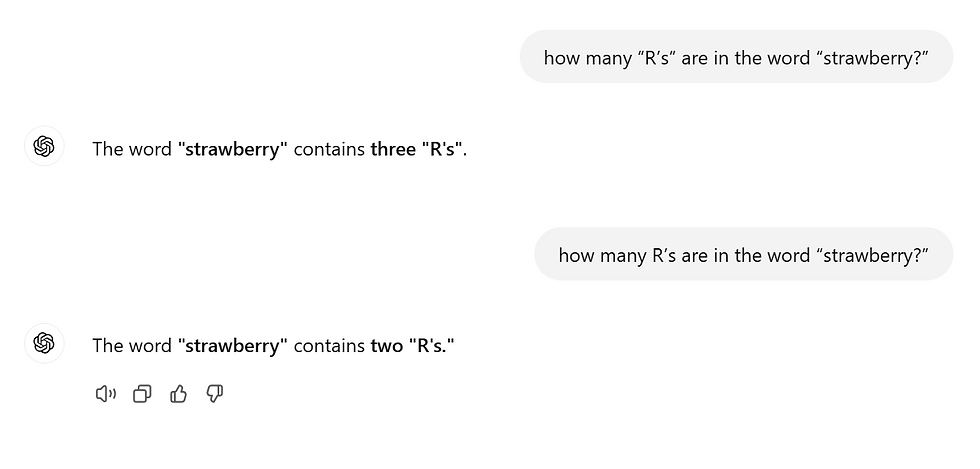
I’m going to ask a very simple question to you: how many “R’s” are in the word “strawberry?” I’m sure you said three. Now, I’m going to ask the same question to ChatGPT, a well-performing large language model that has written complex essays for people and answers various questions. As you can see in the cover photo, it said three and then two. Ok, is this really the high-performing algorithm everyone thinks it is?
Machine learning has potential. Its ability to analyze data and recognize patterns is revolutionary. Nowhere has this potential been more promising than the realm of medicine and healthcare -- from predicting outcomes to identifying disease markers, machine learning holds promise for reshaping how we approach health. However, machine learning is flawed. It face limitations that stem from the inherent way it works.
Ok, so before diving into the limitations of machine learning, let’s talk about the strawberry problem.
Before any large language model received the input we typed into it, it first tokenizes it, meaning converting every word or every part of a word to a token ID which converts it to a series of digits. The prediction the AI model makes is based on these particular digits - not the actual text input. The computer never sees the word, nor parses through it to find the letter R in the word strawberry. It just makes, well, a guess! (And I’d like to give credits to this youtube short https://www.youtube.com/shorts/7pQrMAekdn4 for inspiring this hook to today’s blog post).
Similar to this laughable failure of most large-language models, some AI models can trip up on problems both simple and complex. But, why is that?
The first thing I want to highlight is the success of machine learning models hinged entirely on the quality (and quantity) of the data they’re trained on. This is a limitation. Let’s say a biased programmer only shows the model data from one part of the world. This would make the model unusable, inaccurate, and possibly dangerous if individuals from other parts of the world employ the model for their own applications. In the context of healthcare, this becomes an even more critical limitation. Medical datasets are frequently incomplete or biased. For example, electronic health records (EHRs) are rich sources of patient data but are notoriously messy, with missing fields, non-standardized inputs, and inconsistent coding practices.
Moreover, high-quality labeled data—essential for supervised learning (and if you want a review of what that is, check out: https://www.lavinthelab.com/post/supervised-vs-unsupervised-ml ) —is hard to come by in medicine. Annotating medical images or patient records requires significant time and expertise, often involving highly trained specialists. This limits the diversity and scale of available datasets, making it difficult to generalize ML models across different populations, geographies, and healthcare settings. As I mentioned earlier, location is a common limitation. For example, a model trained on data from one hospital or demographic may perform poorly when applied to a different patient population due to variations in care practices, genetic factors, or socioeconomic conditions.
Bias is an inherent risk in machine learning, and in healthcare, its consequences can be particularly dire. If an ML model is trained on biased data, it is likely to perpetuate or even amplify existing health disparities. For example, a model trained predominantly on data from urban, affluent populations may underperform when applied to underserved rural communities or minority groups.
Bias in healthcare data can arise from systemic inequities, such as unequal access to medical care, historical underrepresentation of certain groups in clinical trials, or socioeconomic factors that influence health outcomes. When ML models fail to account for these biases (mainly because their programmer failed to account for these biases), they risk making inaccurate predictions or recommendations for marginalized populations, exacerbating rather than addressing health inequities.
Also, even if the dataset is well-created and avoids bias, the ethical implications of machine learning can’t be overstated. Patient data is sensitive, and ensuring its privacy and security is a significant challenge. The use of ML in healthcare often involves aggregating data from multiple sources, increasing the risk of data breaches or misuse. High-profile cases of healthcare data leaks have raised concerns about the adequacy of existing safeguards and the potential for patient harm.
In the future, even if these limitations behind machine learning algorithms are fixed, it’s not an easy road up ahead. The deployment of machine learning in healthcare is subject to strict scrutiny.
Agencies like the FDA in the United States require rigorous testing and validation of medical devices and software to ensure safety and efficacy. However, the iterative nature of ML models—which often require continual updates as new data becomes available—complicates this process. Each update may necessitate additional regulatory approval, creating a significant bottleneck.
Furthermore, legal frameworks for liability in machine learning-driven healthcare decisions remain underdeveloped. If an ML model provides an incorrect prediction that leads to patient harm, who should be held accountable: the developer, the clinician, or the healthcare institution? These unresolved legal questions create additional barriers to adoption.
Machine learning holds promise for transforming healthcare. But it doesn’t take a rocket scientist to see its limitations, whether by doing research on why machine learning hasn’t been adapted for healthcare or by asking ChatGPT a simple question. We can’t pretend these problems don’t exist; avoiding a problem doesn’t fix it. Instead, being aware of these challenges and addressing these limitations will help us fix them and improve healthcare simultaneously in the future.
Comments